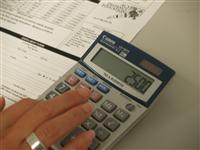
Statistical data is used for better management in every industry in our society. It is critical for conducting scientific research, and vital to managing finance.
A knowledge of statistics can help you at work, in your studies, and in your private life. A lack of understanding at least basic statistics, can be a disadvantage in today's workplace.
This course may not make you into a statistician, but it will provide the most essential knowledge and skills required by consultants, researchers and managers across a wide variety of disciplines.